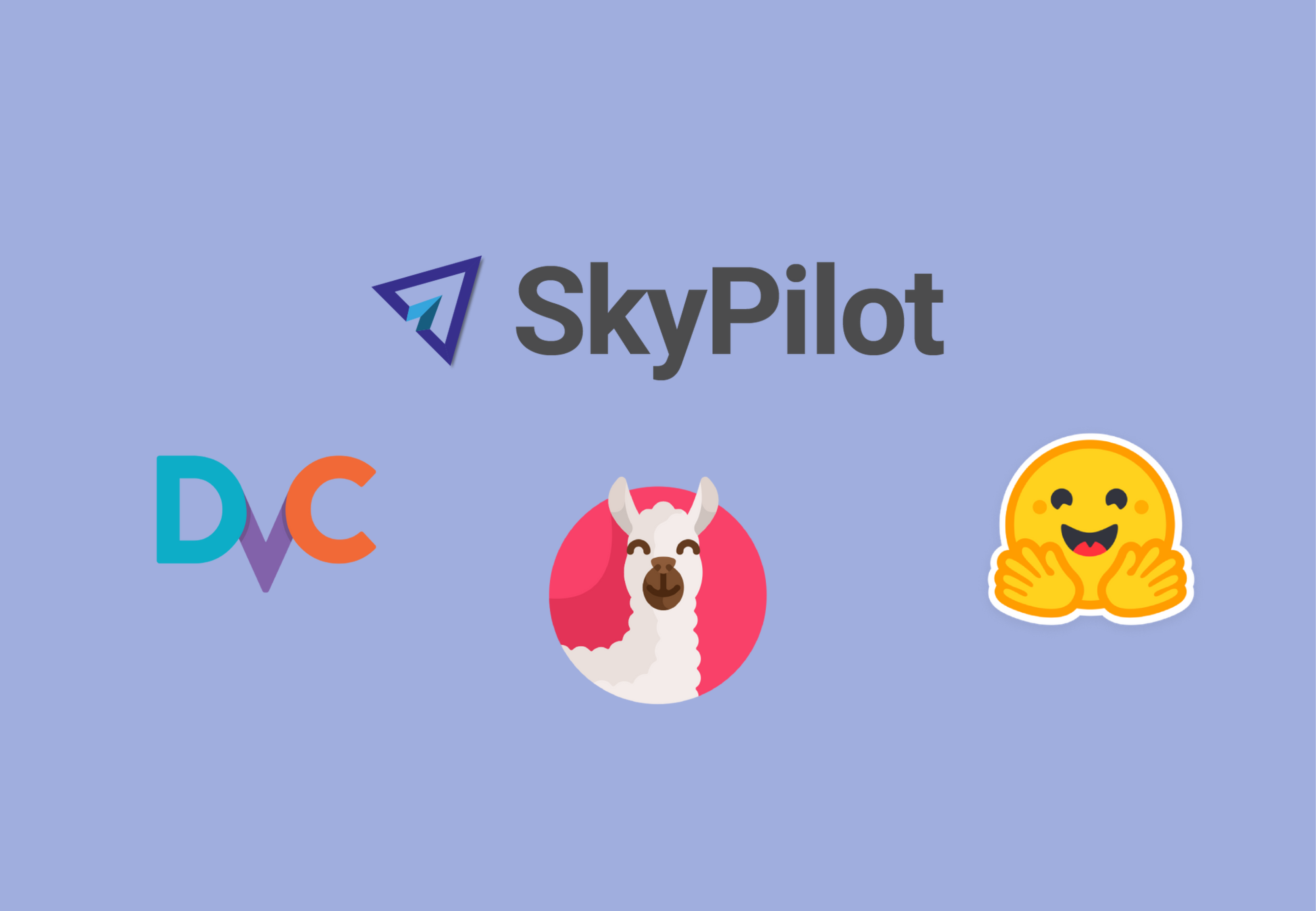
Fine-Tuning Large Language Models with a Production-Grade Pipeline
Introduction - Solving cloud resources and reproducibility for LLMs A few of weeks ago, I wrote a post about the challenges of training large ML models, in particular: the need for more computing power and the complexity of managing cloud resources; the difficulty of keeping track of ML experiments and reproducing results. There I proposed a solution to these problems by using SkyPilot and DVC to manage cloud resources and track experiments, respectively....